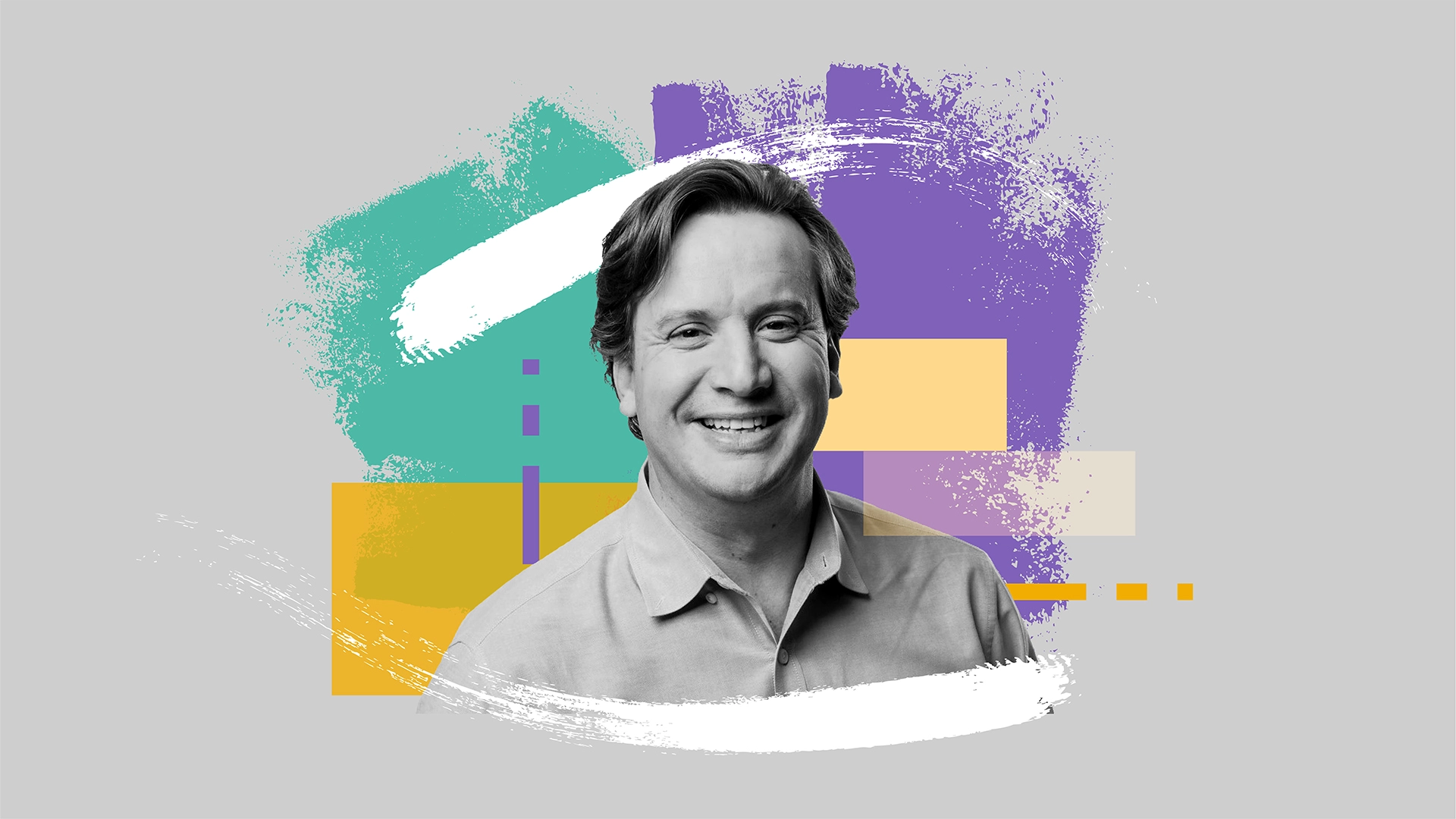
“With artificial intelligence and data at our fingertips, we now have the tools to address the world’s most pressing problems. We no longer have excuses.” That’s the message of Microsoft Corporate Vice President and Chief Data Scientist of our AI for Good Lab Juan Lavista Ferres. He leads a team of data scientists and researchers who partner with organizations like the UN, the American Red Cross, and the Nature Conservancy to help them understand how to use AI to further their humanitarian missions.
Lavista Ferres recently co-authored the book AI for Good: Applications in Sustainability, Humanitarian Action, and Health. He joined us on the podcast to share key insights from the book and highlight what business leaders can learn from the lab’s efforts to harness technology to make the world a better place.
Four big takeaways from the conversation:
AI is a huge unlock for data at any organization. Lavista Ferres says he realized the impact that AI could have on data science when he was telling medical researchers how algorithms could help them extract new insights from vast amounts of CDC data. “A lot of organizations have a significant amount of unstructured data, whether it’s images or video or text, and until very recently that was very difficult to work with,” he says. “Now, thanks to large language model AI, that is changing. Suddenly we have a new tool in our toolbox and we are able to solve problems we couldn’t solve before.”
AI can be a great leveler. “I think these AI models have a huge potential to help with the digital divide,” Lavista Ferres says. “In many ways, human-computer interaction will become much easier, much more natural, and that is going to change the way a lot of people live and work.” But, he notes, to use the technology you need to be able to get your hands on it. “This technology is great as long as you have access. So I think that one of the critical aspects for the world is to ensure that we provide everyone with these tools.”
AI is a game changer for accessibility. “I’m not a native speaker of English, but when you’re working in an organization or publishing research, you are expected to have very good English,” Lavista Ferres says. He explains that AI is an invaluable editing tool that he uses on a daily basis, and that’s just the tip of the iceberg in terms of the impact that AI is having on accessibility. “I think anybody that wants to know how AI is changing the world should talk to people with disabilities,” he says. “There are 1.3 billion people who live with disabilities, and I would say this is really a huge game changer for a lot of those communities.” He cites as an example vision-impaired friends of his who are using AI to help them parse their surroundings and navigate the world.
Nonprofits need AI at work for the same reasons all organizations do. While the AI for Good Lab assists organizations with disaster response projects, climate change initiatives, and healthcare research, Lavista Ferres stresses that a key way in which AI can aid them is by helping with the same sort of resource-intensive day-to-day tasks most companies face. “We need to make sure that every single person at a nonprofit is as productive as possible,” he says. “My wife runs a nonprofit bilingual school, and from reviewing documents to sending emails to applying for grants, these tools help them a lot.”
WorkLab is a place for experts to share their insights and opinions. As students of the future of work, Microsoft values inputs from a diverse set of voices. That said, the opinions and findings of the experts we interview are their own and do not reflect Microsoft’s own research or opinions.
Follow the show on Apple Podcasts, Spotify, or wherever you get your podcasts.
Here’s a transcript of the conversation.
MOLLY WOOD: This is WorkLab, the podcast from Microsoft. I’m your host, Molly Wood. On WorkLab we hear from experts about the future of work, from how to use AI effectively to what it takes to thrive in the digital age. Today I’m talking with Juan Lavista Ferres, Microsoft Chief Data Scientist and the director of the AI for Good Lab at Microsoft. Lavista Ferres co-founded the lab in 2018 and leads its team of dedicated data scientists and researchers who use AI to help address challenges around the globe, working especially closely with government agencies and nonprofits. In this episode, we discuss how AI is being applied to everything from increasing biodiversity to preserving cultural artifacts to medical research. Juan also shares some responsible AI practices and the business value of AI adoption. Please note that our conversation does veer into some heavy topics, including Sudden Infant Death Syndrome. Here’s my conversation with Lavista Ferres.
MOLLY WOOD: Juan, thanks so much for joining me.
JUAN LAVISTA FERRES: Thank you, Molly, for the invitation.
MOLLY WOOD: So when you think about the reason you have the Microsoft AI for Good Lab, what would you say is the high-level mission?
JUAN LAVISTA FERRES: So our mission is to help the world with AI, help organizations around the world on some of the world’s greatest challenges. We are not experts on the problems that we’re solving. Our expertise is in AI. And the reason why it’s important, today, a majority of the expertise works in the financial sector or in the tech industry. The organizations that work with us across the world, these organizations typically do not have the structural capacity to hire the AI talent that is needed to solve this problem—not to hire, not to attract, not to retain. And that’s why, for us, it’s so critical, like, we believe that by donating our time it would make a bigger impact than just a philanthropic donation, and hope that some of these organizations could hire, because it’s going to be difficult for them to hire. So we’re trying to fill that gap, and along those lines try to help these researchers understand how they can use AI and do a knowledge transfer to them.
MOLLY WOOD: And as the capabilities of large language model AI expand, are you widening the aperture of ways you offer help to these organizations?
JUAN LAVISTA FERRES: With large language models, we are now being able to solve problems we couldn’t solve before. A lot of the data, a lot of the problems—whenever, like, organizations store data, a significant amount of data is unstructured data, whether it’s images or video or text. And until very recently, specifically text, that was a very difficult problem to solve. And even if the information was in text, it didn’t mean that you could do something with it. Now, thanks to large language models, that is changing because suddenly you have a new tool in your toolbox.
MOLLY WOOD: Tell us how you first started to see that potential in data science and AI.
JUAN LAVISTA FERRES: Before coming to Microsoft, I used to work in the Inter-American Development Bank, and part of my job was to evaluate projects, and these projects can expand from health to water and sanitation, with a focus in Latin America and developing countries. And that’s the first time that I saw how technology could potentially help these countries and organizations within those countries. Then I moved to Microsoft. I started working in Bing, I worked with Xbox, with Windows, and at one point in my career, a person very dear to me had lost a child to SIDS. SIDS is Sudden Infant Death Syndrome, and it’s the number one cause of death of babies in the US between one month and one year old. And, basically, SIDS is when your baby dies and doctors don’t know why. He was doing an amazing job raising awareness. I asked, I would love to see if we could help, not just with raising awareness, but could we actually help on the data science side? And that was kind of a crazy idea, but he put us in contact with the people at Seattle Children’s Hospital. We found online, there’s an open data set that the CDC has. It’s a data set that has every single baby that has been born, that was born in the US, for the last more than 20, 30 years. And it’s a cohort of those babies that died before one year. Using that data, we were able to find some insights about SIDS, and then we share those insights with these doctors. This is just basically using AI algorithms on top of that data. A lot of these insights, these doctors were aware, but some of the insights the doctors were not aware, and immediately after talking to these doctors, we realized two or three things. The first one is, these researchers didn’t have enough knowledge to work with the data that we were using. So just helping them, and this is not a huge data set, you have 4 million babies that are born in the US every year. So like 10 years’ worth of data is 40 million rows. So it wasn’t a huge data set, but it was difficult enough for them to work with it. But more important, they were not aware too much about the algorithms that we would be using. So they immediately saw a lot of value. And that started this relationship, this collaboration, between us and these doctors about SIDS. And at one point we were invited to share this with Satya and with Brad.
MOLLY WOOD: Satya Nadella and Brad Smith, I should say, the CEO and president of Microsoft.
JUAN LAVISTA FERRES: Correct. Yes. And they saw the value of the things that we were doing.
MOLLY WOOD: And then, what is your day-to-day job at the AI for Good Lab?
JUAN LAVISTA FERRES: My background is the combination between healthcare and AI. So I usually tend to work a lot in healthcare-related projects, but some of my favorite projects that I have done over the years myself has been on giraffes, which are very dear to me. We still work with this amazing organization out of Tanzania, and basically it’s using AI models to identify—this is not just identifying a giraffe, this is identifying giraffe number 45. How is this giraffe related from a social network, like, giraffes live in social networks. How have these social networks changed over time? What is the difference between genders on giraffes? And this information is critical to understand for conservation efforts.
MOLLY WOOD: Okay, first of all, giraffes are my favorite animal, so thank you for doing that. And I want to hear more about that idea of tech transfer, knowledge transfer. I know that’s central to what you wrote about in the book you recently released, right? It’s called AI for Good: Applications in Sustainability, Humanitarian Action, and Health.
JUAN LAVISTA FERRES: Yeah, so we started thinking about the book because anytime that we wanted to work with teams, teams on the ground, it was difficult to explain what they could do with AI. But one recipe that worked really well for us is, we wanted to showcase what other problems we were solving, even if these problems have nothing to do with the type of projects that they had. It was useful for them to understand what else the tool can do, correct? To give you an example, one of the early projects was working with NOAA on detecting and tracking beluga whales underwater in Alaska.
MOLLY WOOD: Let me jump in here, that’s NOAA, the National Oceanic and Atmospheric Administration.
JUAN LAVISTA FERRES: Yes, that is an AI project where you get acoustic data and you try to find a particular beluga whale. When working with another organization out of California, their job was to help on trying to find war crimes. They asked, when we showed that example, Could you use this for detecting a certain type of weapon that makes a very distinct sound? And basically we told them, well, if it makes a very distinct sound and you have these in recordings, they have millions of videos, the answer is likely yes. Because these problems are basically the same problem. You have what is called an acoustic fingerprint. Long story short, it became really easy for us to explain AI by example. And these examples have a lot of variants. Like, you go from projects about disaster response. You have projects on climate change, for example, on trying to measure how climate change is affecting the Himalayas and how dangerous that could be. You have these lakes on top of the mountains that if they don’t, like, they could actually go down and that could kill people, basically. So, this organization out of Nepal uses these models to measure these.
MOLLY WOOD: Okay, so far you’ve covered pretty much two of my three favorite animals in giraffes and whales, and if you say that you’re also working on hummingbirds, I’m going to apply for a job at your lab...
JUAN LAVISTA FERRES: We are working with a lot of birds in the Amazon, that includes hummingbirds...
MOLLY WOOD: I will have my resume in your inbox by the end of the day. I know also AI for Good is a broad remit, and can you tell us how you’ve also applied it to arts and culture?
JUAN LAVISTA FERRES: Yeah, so, AI is very broad. It can, as a general purpose technology, can be used for many things. So one project that we did was a collaboration between Microsoft and Iconem, that is a company out of France, and the French government, was to, on the anniversary, the 80th anniversary for D-Day, was to use vision models to do a description of the pictures. Also leverage a large language model to make searches. This was a website that we launched. And this information could help historians. It also could help people that wanted to learn more about the D-Day. We are working on a few other projects. One of the best scenarios for, if you ask me, for cultural heritage, is the power of vision models to make descriptions, particularly for blind people. This has been used in museums now. And we are using for a few other projects where, given a picture or given even a video, you can make a very accurate description of what you see there. That is certainly a game changer for a lot of these low-vision and blind individuals.
MOLLY WOOD: Clearly there is tech transfer and knowledge transfer and value in the work itself. And also it seems like there must be some extrapolations from a business lens about how to make do with limited resources, right? This is the situation that nonprofits are always in, but many businesses are too. I wonder if you can talk about what learnings you’ve gotten.
JUAN LAVISTA FERRES: I think in general, a lot of the problems that we work with nonprofits are problems that could be working, like you said, in multiple industries. When we see the same problem being asked by multiple organizations, we try to focus on those projects. And let me give you a great example of that. That is our disaster assessment tools. Whenever there is a natural disaster, a lot of organizations need to have an understanding of what is happening on the ground. How many people were affected? Where are those people affected? And when we talked to multiple organizations, like from UN agencies to the International Organization for Migration, to American Red Cross, to different Red Crosses across the world, everybody was looking for something like that. That’s why we decided to say, hey, this is going to be a pillar for us. This is going to be an area of investment. Let’s build tools. So we’re not just at the beginning, we are going to help you do these disaster assessment maps, but ideally we will give you the tools so you can do it yourself. And that’s an area that for us has been an area of priority. So we work with these organizations on the ground and we provide them with these disaster assessment AI models to generate disaster assessment maps.
MOLLY WOOD: One of the central tenets of doing good is also mitigating harm or avoiding harm. I want to ask you about AI responsibility and how you define and think about responsible AI.
JUAN LAVISTA FERRES: Responsible AI is at the core of the projects we do. And this is also a place where I think Microsoft was much ahead of other organizations. And this is, for the last five years, we have our Office of Responsible AI. We have Natasha Crampton, who’s our Chief Responsible AI Officer, does an amazing job and has an amazing team try to help us, not just us, but multiple teams across Microsoft and even influence the industry in many ways on how we can use AI in a responsible way. So for every project we have, it goes through a responsible AI process to try to make sure that we mitigate as much as possible any potential harms from these models. When we’re working with, for example, people that are losing their voice through degenerative diseases like ALS. When you work with them, you realize that their tone of voice that eventually they will lose. And, eventually, they will use machines to speak. But the tone of voice is critical to their identity. It’s very important. And thanks to AI, thanks to generative AI models today, you can clone a person’s voice and you can use a machine that will speak on your same tone of voice, which is a game changer for people that suffer from these diseases. But at the same time, you can use the same technology to clone someone else’s voice and do scams. And that is also happening today. Of course, if you want to use some of this technology, Microsoft is really restrictive in that technology for good reasons, because that technology could be used for bad purposes, particularly scamming.
MOLLY WOOD: In your book, you talk about how AI can better analyze data without human bias and remedy pattern recognition deficits, which also seems key to sort of imagining these unintended consequences. Can you give us some examples of how that works?
JUAN LAVISTA FERRES: Bias is a great issue and it’s something that as a society we need to make sure that we address. There’s different types of biases. There was a study that was published a few years ago, it was published in the New England Journal of Medicine. That is the most prestigious medical journal in the world. And what they found was, they took a random sample of people in California that died and asked their family members whether they were left-handed or right-handed. And what they found, what the researchers found, was that left-handed people were dying nine years younger than right-handed people. This is really disturbing. Like, that’s the equivalent of smoking 120 cigarettes per day. And the study claimed that the issue, the reason why this was happening is because we live in a world that is made for right-handed people, not for left-handed people, whether you’re driving, or the tools, and that’s why these individuals were dying nine years younger. What the researchers didn’t fully realize is that for a long period of time, there was a discrimination against left-handed people because parents would force their kids to be right-handed. I know that because my grandfather was one of them. He was forced to be right-handed. Eventually, they stopped doing that, and this generated this artificial increase in the left-handed population to the right level, that is roughly 10 percent. So 10 percent of the population is left-handed. But if you look at 1920s, 1950s, 1930s, those numbers were like 3 percent, 3.5 percent. So that generated this artificial increase, this artificial increase is the one that gives us the illusion that left-handed people die younger, when in reality, that’s not the case. The challenge from an AI perspective is that if you have a life insurance company, and you have that data set, and one of your features in the data set is if the person is left-handed or right-handed, what the model will tell you is that you need to charge more to the left-handed people because they will die younger, when in reality that’s not the case.
MOLLY WOOD: Right.
JUAN LAVISTA FERRES: So, a majority of the data we collect has some biases. It’s critical to understand those biases to make sure that we don’t perpetuate those biases. Not all the biases are generated by changes in culture, like the left-handed. Some type of biases could happen just because we have an unconscious bias in the way we hire. There was another example a few years ago where a company decided to use AI models to do the screening process in HR. And even though gender was not one of the features, the model learned that the chances of being hired was affected by gender because that was some of the behaviors of that company before. And the problem is that once you train a model with that data, the model will perpetuate that bias and will just continue. So we need to understand that the data that we’re using to train AI models is the code of that model. So if the data has issues because it has some bias, the model will learn those biases and will perpetuate those biases. And working to solve bias is not an easy problem. In some cases we can at least detect it and try to work with it, but it’s not an easy problem.
MOLLY WOOD: I want to switch gears a little bit. WorkLab is, of course, a podcast for business leaders who want to get a handle on how work is changing. And it feels to me like what the AI for Good Lab is doing also lets those business leaders think maybe more creatively about how to deploy and use AI in their organizations, and I wonder if you can speak to that based on the experiences you’ve had. How can AI help people grapple with the bigger challenges they face?
JUAN LAVISTA FERRES: Yeah, again, I think the book describes that in the sense that like a lot of the examples that we have could be used for other purposes. The techniques we use, like computer vision techniques, they can be applied for multiple scenarios in different industries. Even, for example, the disaster assessment tools. So every time there’s a big natural disaster, we use these disaster assessment tools to build the maps and share these maps with organizations on the ground. Even insurance companies have reached out to us, saying, Hey, could we use that same technology? We don’t work with those companies, but they are solving the same problem, basically. So I would say, in general, the answer is yes. I would say a majority of the programs that we work for, these nonprofit organizations, could be applied to other areas.
MOLLY WOOD: I grew up in and around nonprofits. This is the work that my mom did my whole life and, like any business, the backend, the operations of things are really crucial. And sometimes you have organizations that are understaffed, they’re underfunded, and it feels to me like a key component of being able to use AI to do good at a nonprofit is, frankly, the simple ability to make better spreadsheets, to operate more efficiently, to have summaries of emails to just move more quickly in the world. Has that been your experience?
JUAN LAVISTA FERRES: That is definitely my experience. And there’s a whole group in Microsoft that works specifically in those scenarios. This is the Tech for Social Impact that is also within Microsoft philanthropies. They do an amazing job helping on some of those scenarios. And like you said, this is particularly affecting the nonprofits where every single person, we need to make sure that they’re as productive as possible. A lot of these scenarios, from reviewing to sending emails to—my wife runs a nonprofit, she runs a bilingual school, and from communications to notifications to applying for grants, these tools help them a lot. So yes, the answer is yes. There’s a whole group in Microsoft, like a lot of folks in a lot of those scenarios that’s, like I mentioned, Microsoft Tech for Social Impact.
MOLLY WOOD: What is next for the lab? What are you most excited about?
JUAN LAVISTA FERRES: So we’ve been working a lot in the Amazon. We’re going to be in Cali, Colombia, for [the UN] COP Biodiversity [Conference]. And we are working with organizations, nonprofit organizations, and some government agencies in Colombia to use our models to measure and sometimes even alert on potential deforestation. Deforestation is something that’s critical for the Amazon, it’s critical for Colombia, it’s critical for any, all the countries that are within the Amazon. So we want to make it easy for these countries to be able to measure deforestation and to detect deforestation.
MOLLY WOOD: Okay, I want to ask you before I let you go a couple of lightning round, quick questions. How do you use AI yourself, at work or in your personal life?
JUAN LAVISTA FERRES: So I use AI every day for doing our job in many ways. But for me, what has been a game changer, particularly in large language models, have been the ability to edit my English, as you likely realize by my perfect English accent, I’m not a native speaker of English. So when you’re either publishing or you’re working in an organization, it’s expected to have very good English. And it would take a lot of effort for me to edit my English. And I think in many ways, large language models are helping me a lot on that end. I use it a lot for research, for helping to find things. I think it’s a great research assistant. It sometimes makes a mistake, and that’s something that we always need to be conscious about, but it’s an amazing tool that can help on the research side. And yes, I’m using it more and more, I would say.
MOLLY WOOD: In your experience, what is the use case for AI that seems to be the biggest unlock for people that really gives them kind of an aha moment?
JUAN LAVISTA FERRES: I think there’s a lot of scenarios, but having friends and working with people with disabilities, I think this technology is a true game changer. I have friends that are blind that are using vision models to help them navigate the world and help them understand and see pictures or see where they are, to help them with their life. And I think anybody that wants to know how AI is changing the world should talk with people with disabilities. We live in a world where 1.3 billion people suffer from disabilities. And I would say for a lot of those communities, this is really a huge game change. I’m also very passionate, like I mentioned, about healthcare. I think that there’s a huge potential on how we can use this technology to help better understand the disease and the diagnostics.
MOLLY WOOD: And then finally, if you wouldn’t mind, fast forward 3 to 5 years. And what do you think will be the most profound change in the way we work?
JUAN LAVISTA FERRES: It’s difficult to talk about the future in many ways. But I think these AI models will help us, have the huge potential to help with the digital divide in many ways. It can also exacerbate for those people that do not have access to the technology, and this is something that, like, the human computer interaction will become much easier, much more natural. And that is something that is going to change the way a lot of people live and work. I am concerned that in order to use this technology, you first need to have access to electricity. We live in a world where 750 million people do not have access to electricity. You actually have to be connected. You have 2.3 billion people that are not connected. So I’m concerned that this technology is great as long as you have access. So, I think that one of the critical aspects of the world is to make sure that we provide them the tools for having that accessibility.
MOLLY WOOD: Thank you again to Juan Lavista Ferres, Microsoft Chief Data Scientist and the director of the AI for Good Lab at Microsoft. I really appreciate the time.
JUAN LAVISTA FERRES: Thank you very much, Molly.
[Music]
MOLLY WOOD: Please subscribe if you have not already, and check back for the rest of season 7, where we will continue to explore how AI is transforming every aspect of how we work. If you’ve got a question or a comment, please drop us an email at worklab@microsoft.com, and check out Microsoft’s Work Trend Indexes and the WorkLab digital publication, where you’ll find all our episodes, along with thoughtful stories that explore how business leaders are thriving in today’s new world of work. You can find all of it at microsoft.com/worklab. As for this podcast, please, if you don’t mind, rate us, review us, and follow us wherever you listen. It helps us out a ton. The WorkLab podcast is a place for experts to share their insights and opinions. As students of the future of work, Microsoft values inputs from a diverse set of voices. That said, the opinions and findings of our guests are their own, and they may not necessarily reflect Microsoft’s own research or positions. WorkLab is produced by Microsoft with Godfrey Dadich Partners and Reasonable Volume. I’m your host, Molly Wood. Sharon Kallander and Matthew Duncan produced this podcast. Jessica Voelker is the WorkLab editor.
More Episodes
Subscribe

WorkLab Guest Timm Chiusano
Timm Chiusano on AI and “Winning the Week”
The TikTok superstar and former creative executive shares his unique career journey and leadership insights.
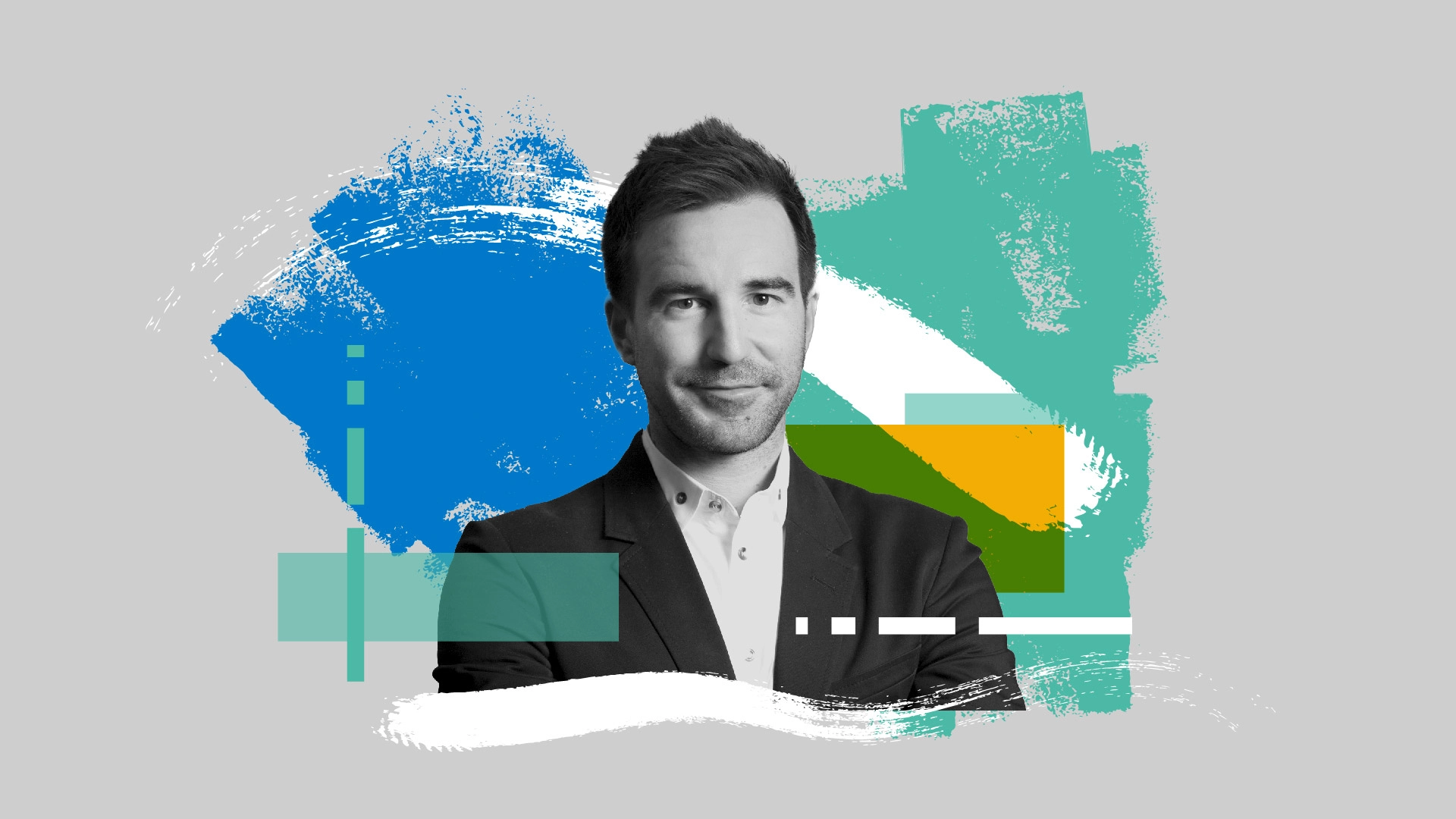
WorkLab Guest Tomas Chamorro-Premuzic
How Can Leaders Invest the Time That AI Gives Back?
Psychologist and author Tomas Chamorro-Premuzic explains how AI can unlock greater performance.