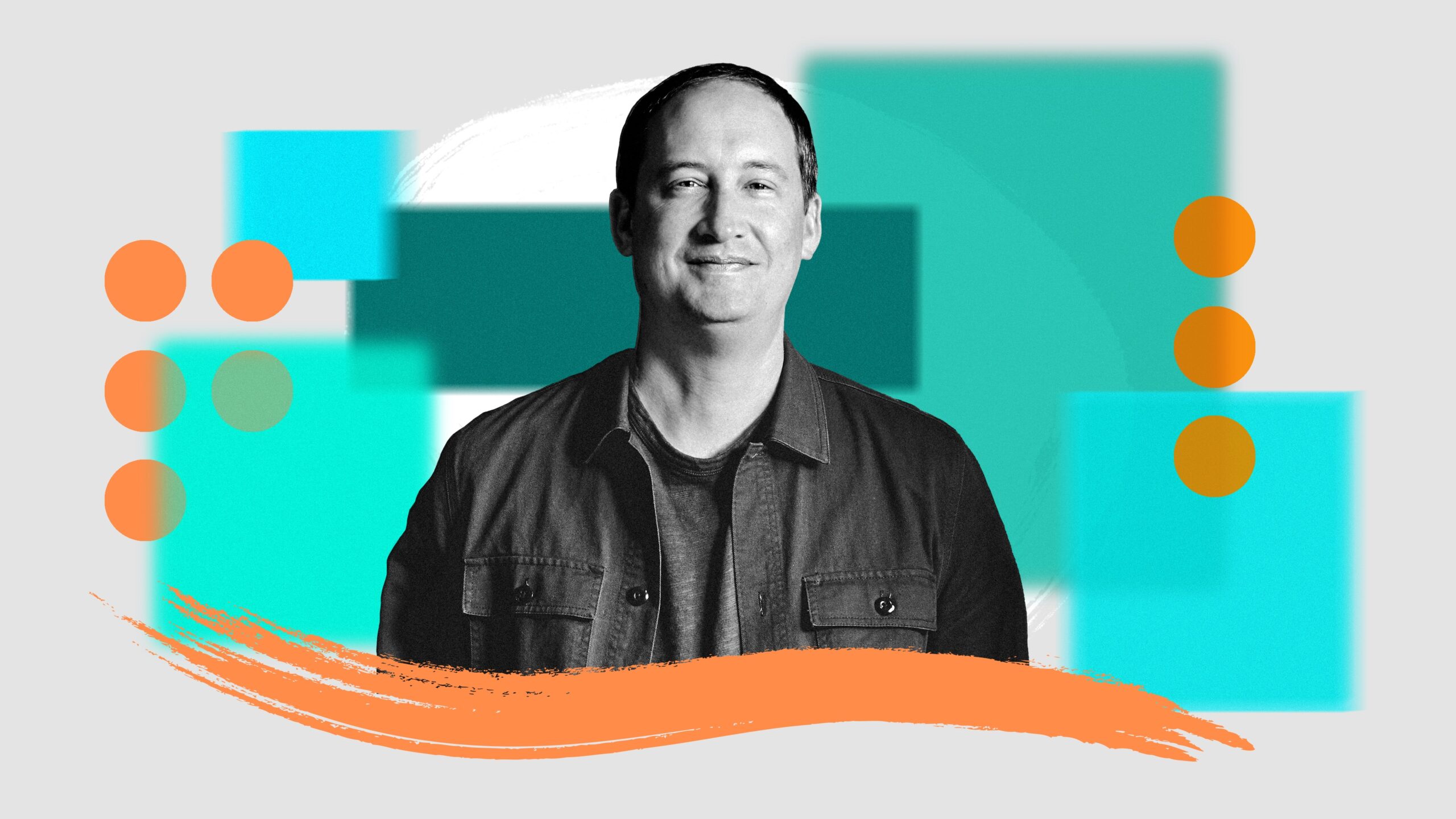
New innovations in AI sound exciting, but how will they actually change the way we work? Marcus Wohlsen is here to share some insights. Wohlsen is a journalist, author, and the head of editorial at the storytelling firm Godfrey Dadich Partners, and he has a special expertise on the past and future of AI. He provides a unique perspective—and some much-needed context—to help us as we try to wrap our heads around how AI will transform the future of work.
Wohlsen is the fourth guest of season 4 of Microsoft’s WorkLab podcast, in which host Elise Hu has conversations with economists, designers, psychologists, and technologists who explore the data and insights into why and how work is changing.
Three big takeaways from this conversation:
“I’ve been thinking about AI as this kind of relevance engine,” Wohlsen says. “It has this amazing ability to personalize the information that we consume, and that’s because we can talk with it in the way that we talk with one another.” He gives a hypothetical example: “Let’s say you’ve been on vacation for a week and your inbox is stuffed with hundreds of emails. Imagine being able to just ask the AI agent to go through that inbox and pull out the action steps you need to take. Or imagine being able to just say, what’s the status of this particular project?”
AI can do your work for you, but it can also help you get past the terror of a blank page by quickly generating a raw initial draft that can serve as a valuable jump-start. “One of the things that’s going to start to become really pervasive as AI becomes more widespread is that we probably aren’t going to start with a blank page,” Wohlsen says. “You can simply pose a question and the AI tool will give you an answer. It might not be the right answer, but you’re going to have something there to start with.”
We will always need to have a human scrutinizing the outputs of AI and using their judgment to make sure it’s accurate and useful. “A machine can simulate that kind of judgment, but it’s just running probabilities and making predictions based on data that comes from us,” Wohlsen says. “We’re feeding these machines with information that it’s giving back. It’s still on us to figure out whether what we’re making with these things is any good, whether it matters, whether we need it or not.”
WorkLab is a place for experts to share their insights and opinions. As students of the future of work, Microsoft values inputs from a diverse set of voices. That said, the opinions and findings of the experts we interview are their own and do not reflect Microsoft’s own research or opinions.
Follow the show on Apple Podcasts, Spotify, or wherever you get your podcasts.
Here’s a transcript of the episode 4 conversation.
ELISE HU: This is WorkLab , the podcast from Microsoft. I’m your host, Elise Hu. On WorkLab , we hear from leading thinkers on the future of work. Economists, designers, psychologists, technologists all share surprising data and explore the trends transforming the way we work.
MARCUS WOHLSEN: One of the things that is going to start to become really pervasive as AI becomes more widespread is that we probably aren’t going to start with a blank page. You can simply pose a question, and the AI tool will give you an answer. It might not be the right answer, but you’re going to have something there to start with.
ELISE HU: Marcus Wohlsen is a journalist, author, and head of editorial at the storytelling firm Godfrey Dadich Partners. He has worked with Microsoft and other clients to envision a future shaped by the latest advances in artificial intelligence. He’s here to help us understand how this moment fits into the broader history of AI’s development, and how we can expect AI to change the world of work for all of us.
ELISE HU: Hey, Marcus. Thanks for doing this.
MARCUS WOHLSEN: Hey, Elise. My pleasure.
ELISE HU: You’ve spent a lot of time covering the tech industry and the history of artificial intelligence. What is your sense of what’s happening in this moment?
MARCUS WOHLSEN: As a journalist who has been covering the rise of AI, especially over the last decade, we’re in a moment now of pretty stunning disruption—it’s a word that gets overused, but I think it’s important to recognize it when it’s actually occurring. And I think the way that we know that, in one way, is that these changes and these emerging capabilities of these large language models are happening at a pace that even the most optimistic researchers didn’t predict themselves.
ELISE HU: This all seems so novel and new to us right now, but couldn’t you make the case that all of us have already integrated AI into our everyday lives? Been using it long before these particular developments, right?
MARCUS WOHLSEN: Right. The most useful application of AI in my life, without a doubt, is maps. GPS-based, turn-by-turn direction maps. And what I don’t think we recognize anymore, because it’s so effective and useful and easy, is that every time we ask for directions, a computer is making a prediction about the best way to get there—based on the available data, based on traffic, based on distance, based on speed limits, traffic signals. All of those are data points. And what the AI system is doing in the background is judging probabilities. People spend their time thinking about AI and ask, well, what is AI? Well, it’s anything we can’t quite do yet with machines. When something becomes everyday, like using turn-by-turn directions and GPS-enabled maps, we’re not amazed by that anymore, and it sort of blends in to our everyday lives. What we’re mostly talking about now when we talk about AI, are actually these large language models that are generating these rich textual answers to questions that we pose or to prompts or to requests. Those models are actually still fundamentally operating on the same principle, on a really basic, oversimplified level. Today’s chatbots are predicting based on the prompt that I give it. What’s the word that’s most likely to come next? And it’s basing this on pretty much the biggest dataset of all, which is the entire internet. And so it’s weighing probabilities and spitting out an output. It just so happens that because of a mix of the size of the dataset, unprecedented power of the computing that’s available now, and the sophistication of the models, that probability engine is giving us outputs that start to feel indistinguishable from a human response.
ELISE HU: Marcus, it’s obviously hard to think about how large language model machine learning works without sort of equating it to how the human brain works. Is that why the conversation tends to be on whether AI has achieved sentience, or when it will achieve sentience?
MARCUS WOHLSEN: Right. So it’s very easy to fall into this conversation about whether these large language models are, quote unquote, intelligent. Not that it’s not a question worth considering, but given the speed at which these tools are becoming available to everyone, I think it becomes sort of like a side conversation, because for all intents and purposes, these large language models, they feel intelligent to us. If it feels like there’s a person on the other end of it, I think we’re going to respond to it that way. And so the question really becomes more, okay, now that we have this, what are we going to do with it?
ELISE HU: What are we going to do with it?
MARCUS WOHLSEN: Well, already there are some very practical applications. One of the promises of these large language models of next-generation AI is that they’ll, for instance, be able to summarize meetings—and not just summarize them in kind of a generic way, but each one of us will be able to use these tools to find out specifically what mattered to us. Similarly with onboarding. Onboarding is a process that is really about knowledge gathering and knowledge transmission. The real power of these tools is the ability to have what amounts to a conversation that’s informed by the specific data of my organization. And to be clear, that’s what I’m talking about now, is when you’re putting to use tools like Microsoft’s Copilot tool, the large language models that are out there in general, are primarily pulling from information that’s available on the internet. One of the powerful promises of these in an applied setting is, for instance, in the use of a tool like Copilot, is being able to use the kind of overall ability of these models to interact with us using natural language, but have that interaction being informed by the specific information, by the specific data that is unique to me, that is unique to my organization. Another use case there: Let’s say you’ve been on vacation for a week and you come back to an inbox that’s just stuffed with hundreds of emails and, you know, imagine being able to go into your inbox and just ask the AI agent to pull out the action steps that I need to take, or to say, what’s the status of this particular project? So in the context of work, in the context of knowledge work specifically, I’ve been thinking about AI as this kind of relevance engine. It has this amazing ability to personalize the information that we consume, and that’s because we can talk with it in the way that we talk with one another.
ELISE HU: Well, as a business proposition, let’s just return to the fact that AI is only ever as capable as the data that has fed it. And so what about those who might be hearing this conversation, especially about personalization for workers? What about data privacy?
MARCUS WOHLSEN: Data privacy is a huge issue when it comes to AI. Privacy, issues of consent, issues of data governance—these are all issues that organizations, they’re familiar with them. But it really reaches a whole other level with these large language models. Their usefulness is kind of predicated on the amount and the quality of the data that they consume. But security, privacy, consent, governance—if those aren’t addressed in a very proactive way, it seems like it would be very easy for data to seep into the models where people have access to it who shouldn’t, or people who did not consent to have their data used are finding that it’s been incorporated into them in the first place. So yeah, these are issues that are a big deal right now and issues that leaders and organizations really need to be thinking about very actively.
ELISE HU: Is the way that AI augments our human abilities similar to past technological advancements?
MARCUS WOHLSEN: I think there are some similarities when it comes to augmenting human capabilities. If you think about, say, the calculator, it allowed us to make mathematical calculations faster. If you think about the car, it allowed people to get from one place to another faster and more independently. I think when you look at AI, there is greater efficiency, but it really goes much more to the heart of how we think and how we create. And I think we don’t really know yet what all the potential is there to transform how we do things. But I think that likely there’s a transformation on the horizon that is more profound and fundamental than what some earlier technologies were able to make possible.
ELISE HU: What do you think that looks like, Marcus?
MARCUS WOHLSEN: One of the things that is going to start to become really pervasive as AI becomes more widespread is that we probably aren’t going to start with a blank page in the way that we used to. You know, what do we do? We have a blank page and we need to do some research. So we go online and we do a search and we get a list of web pages and we investigate. Now, already, you can simply pose a question and the AI tool will give you an answer. It might not be the right answer, but you’re going to have something there to start with. I think that, especially for teenagers and younger who aren’t going to really remember the time before these tools were available, it’s going to seem strange to them not to do that.
ELISE HU: Yeah, will we need to learn how to write anymore?
MARCUS WOHLSEN: Right. There is something, I think, something that you lose in a sense if you are simply relying on the machine to do the writing. But more importantly than that is that somebody is always still going to have to evaluate the quality of whatever it is that the machine creates. There are some researchers from the University of Toronto who wrote a great book called Prediction Machines , where they really pose this question of what humans are still going to be necessary for in a world where these systems are as smart as they seem to be now. And what it comes down to is judgment. The machine ultimately still isn’t something that exists in the world in the way that it is able to, quote unquote, know whether this piece of writing is useful, is relevant, is something that we need—is good. A machine can simulate that kind of judgment. But again, it’s still just running these probabilities and making predictions based on data that fundamentally is data that comes from us. This is all us feeding these machines with information that it’s giving back. It’s still on us to figure out whether what we’re making with these things is any good, whether it matters, whether we need it or not.
ELISE HU: What are you most excited about, or what do you find most promising that you’ve seen from the applications?
MARCUS WOHLSEN: I have a colleague who was trying to think through roles and responsibilities in a particular team, and they just asked the AI and the AI shared some ideas. You can take them or leave them, but it gives you a starting point. It gives you a way to kind of kickstart a conversation. I’ve heard of people using AI to create business plans, to create work back schedules. I can tell you a personal story. My son wrote an essay for his English class—and I actually saw him doing some of the writing so I can vouch for the fact he was actually writing it himself. But he fed it to ChatGPT after it was done, and he read back to us what it said, and it gave him an evaluation of the essay. It gave its assessment of what he did well, of providing relevant examples, of providing context, connecting it to personal experience. It said, here are a couple of things that could maybe make it stronger. Oh, and also there are a couple of typos. And in getting that feedback, he learned something, and it also gave him the confidence to turn the essay in because he wasn’t sure if it was good enough. But he thought, basically, after getting that assessment, he was like, yeah, I think this is all right. So it really was really fascinating to me to see that use of AI as this thought partner, as this conversation partner. But I think most importantly, not in a way that’s like substituting for doing the work. It’s not, AI, could you write me this essay and I’m going to cut and paste it and turn it in. What these large language models enable is a new form of interaction with our machines. We can interface with our computers without learning a special language. We can simply interact in the most natural way we know how, which is to use our own voices.
ELISE HU: So beyond the ethical considerations that we talked about a little earlier, what other advice do you want to leave leaders with as we meet this moment for large language models?
MARCUS WOHLSEN: I think for leaders in organizations wrestling with how to make use of it effectively, you really have to appreciate the level of disruption that this represents. Disruption is a word that gets way overused in tech and in business. And so it makes it hard to recognize, I think sometimes, when a real disruption has occurred. I think this is one of them. And so that means needing to have a truly open mind. Leaders themselves need to actually use these tools to see what they’re capable of. You can’t just listen to podcasts about it. You have to do it. And what you also have to do is be comfortable with everybody in your organization using it. The kind of experimentation that’s necessary in order for innovation to happen. It can be challenging, but you’re not really going to be able to grapple with that in an intelligent way unless you try it.
ELISE HU: Well, what an opportunity, too, to get to chart the future. Marcus, thank you so much.
MARCUS WOHLSEN: Great. Thank you.
ELISE HU: Thank you again to Marcus Wohlsen. And that’s it for this episode of WorkLab , the podcast from Microsoft. Please subscribe and check back for the next episode, where we’ll be checking in with Jared Spataro, Microsoft’s Corporate Vice President for Modern Work, on the most important findings and insights from the company’s new Work Trend Index. If you’ve got a question you’d like us to pose to leaders, drop us an email at worklab@microsoft.com, and check out the WorkLab digital publication, where you’ll find transcripts of all our episodes, along with thoughtful stories that explore the ways we work today. You can find all of it at Microsoft.com/WorkLab. As for this podcast, rate us, review, and follow us wherever you listen. It helps us out a lot. The WorkLab podcast is a place for experts to share their insights and opinions. As students of the future of work, Microsoft values inputs from a diverse set of voices. That said, the opinions and findings of our guests are their own, and they may not necessarily reflect Microsoft’s own research or positions. WorkLab is produced by Microsoft with Godfrey Dadich Partners and Reasonable Volume. I’m your host, Elise Hu. My co-host is Mary Melton. Sharon Kallander and Matthew Duncan produced this podcast. Jessica Voelker is the WorkLab editor.
More Episodes
Subscribe
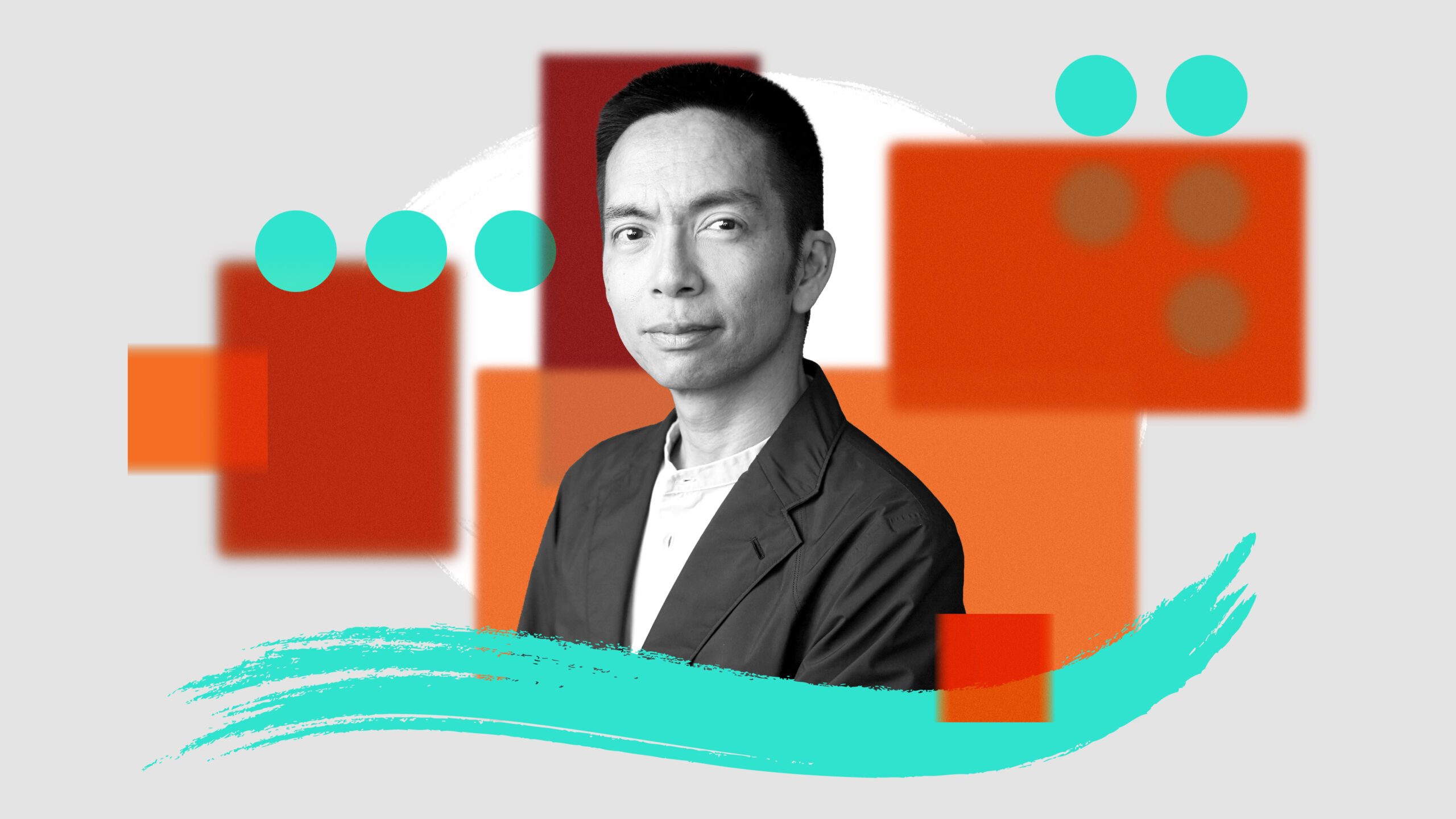
WorkLab Guest John Meada
John Maeda on How Leaders Will Use AI to Unleash Creativity
The Microsoft VP of Design and AI on the promise and potential of AI
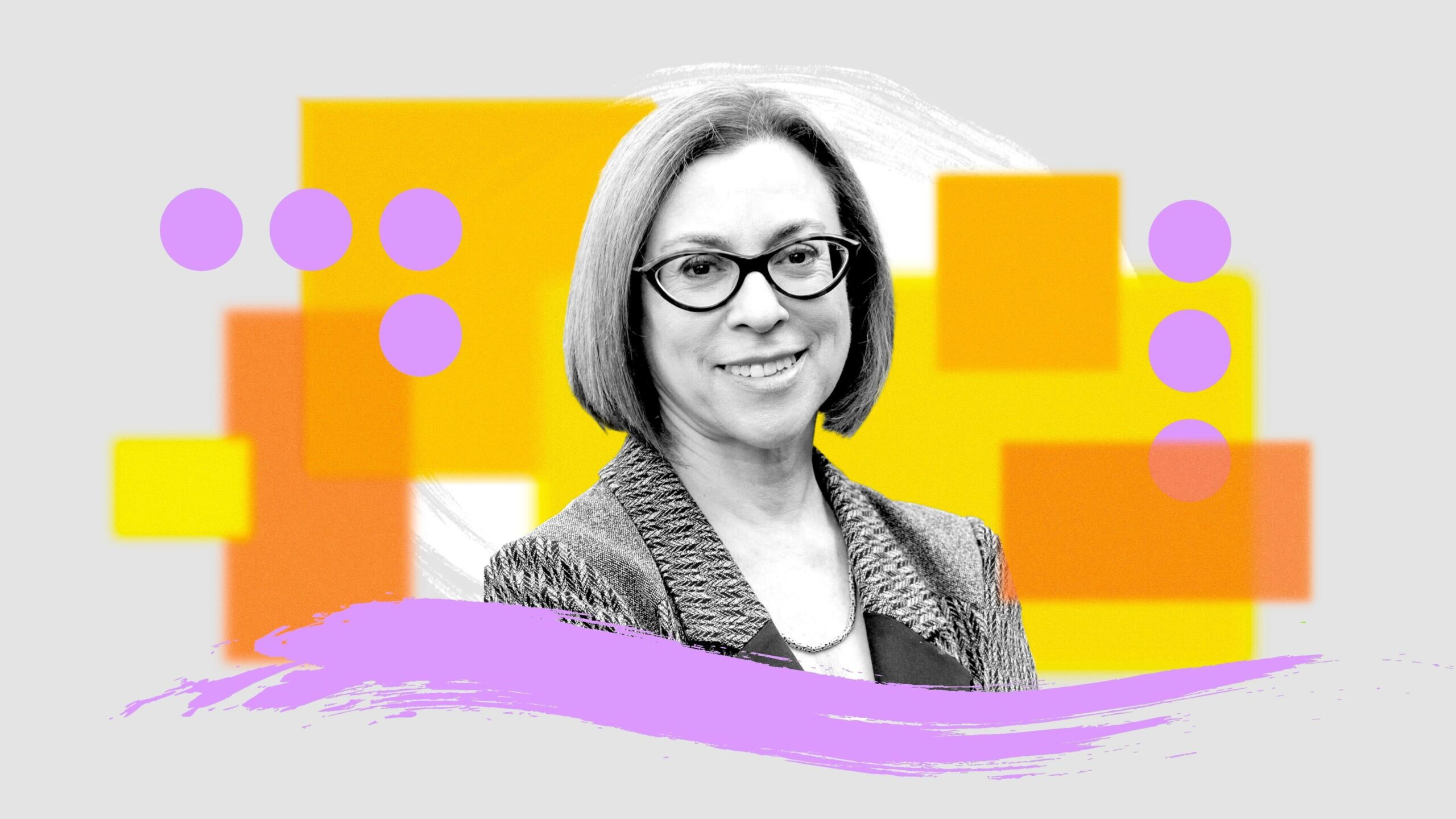
WorkLab Guest Gloria Mark
Regain Control of Your Focus and Attention with Researcher Gloria Mark
The author and professor offers science-based tips for work wellbeing—and a glimpse at how AI might help